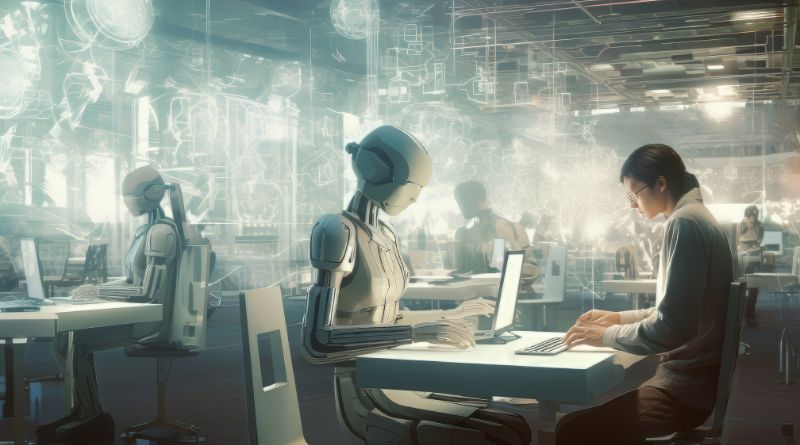
In the ever-evolving landscape of artificial intelligence (AI), the acquisition of high-quality training data has emerged as a critical battleground for tech giants like Google, Facebook, and Amazon. These companies are engaged in a covert but intense race to secure vast amounts of diverse and labeled datasets to fuel their AI algorithms and maintain their competitive edge in the industry.
Understanding the Importance of AI Training Data
AI algorithms heavily rely on large volumes of labeled data to learn and improve their performance. The quality and diversity of training data directly impact the accuracy and effectiveness of AI models. Therefore, possessing comprehensive and well-curated datasets is paramount for companies aiming to develop cutting-edge AI technologies.
Big Tech’s Strategies for Acquiring Training Data
Strategic Partnerships and Acquisitions
Tech giants often forge strategic partnerships or acquire smaller companies specializing in data collection and labeling. By leveraging external expertise and resources, they can rapidly expand their datasets while maintaining high standards of quality and diversity.
In-House Data Generation
Many companies invest in in-house data generation initiatives where they deploy crowdsourcing platforms or develop proprietary tools to collect and label training data internally. This approach provides greater control over the data acquisition process and ensures alignment with specific AI development objectives.
Data Collaboration and Exchange
Collaboration between tech companies, research institutions, and industry partners facilitates the sharing and exchange of training data. By participating in consortiums or open-source initiatives, companies can access a broader range of datasets while contributing to the advancement of AI research and development.
The Ethical and Privacy Implications
While the pursuit of AI training data offers tremendous opportunities for innovation, it also raises significant ethical and privacy concerns. The indiscriminate collection and use of personal data without consent or proper safeguards can lead to privacy violations and algorithmic biases, exacerbating societal inequalities and discrimination.
Conclusion
In conclusion, the race for AI training data underscores the fierce competition and technological advancements driving the AI industry forward. As tech giants vie for supremacy in this clandestine arena, it is imperative to balance innovation with ethical considerations and ensure responsible data practices to foster trust and transparency in AI development. Only by addressing these challenges collectively can we harness the full potential of AI for the benefit of society.