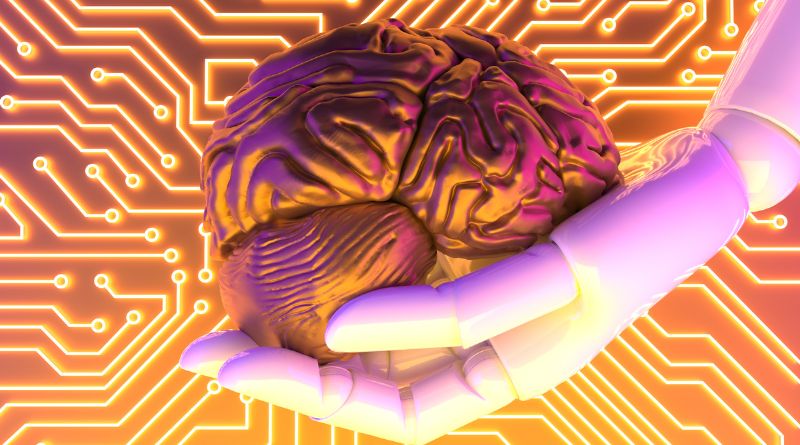
Artificial Intelligence (AI) is reshaping industries, enhancing productivity, and influencing daily life. However, this rapid progress also brings challenges, including biases, inefficiencies, and ethical dilemmas. As AI continues to evolve, Responsible AI emerges as a critical approach to ensure systems operate fairly, transparently, and for the benefit of all.
In this article, we explore how Responsible AI addresses these pressing concerns and creates a foundation for trustworthy, efficient, and inclusive technology.
Understanding Responsible AI
Responsible AI refers to the development and deployment of AI systems that prioritize ethical considerations, fairness, accountability, and transparency. It involves creating frameworks and practices that minimize risks and maximize benefits for society.
Key Pillars of Responsible AI
- Fairness: Eliminating biases to ensure equitable outcomes.
- Transparency: Providing clear explanations for AI decisions.
- Accountability: Establishing mechanisms to identify and rectify errors.
- Ethical Alignment: Ensuring AI systems respect societal values and human rights.
The Challenges of Bias and Inefficiency in AI
Bias in AI Systems
AI systems often learn from historical data that may contain societal biases. When these biases are embedded into models, they can lead to unfair outcomes, such as:
- Discriminatory Hiring Algorithms: Favoring one demographic over another.
- Healthcare Inequities: Providing suboptimal recommendations for certain populations.
- Biased Facial Recognition: Misidentifying individuals based on race or gender.
Inefficiency and Resource Misallocation
AI inefficiencies can manifest as misaligned objectives or excessive resource consumption. For instance:
- Overfitting: Models designed for specific tasks struggle with generalization.
- Energy-Intensive Processes: Training large AI models often consumes significant energy, raising environmental concerns.
How Responsible AI Addresses These Issues
Mitigating Bias
- Diverse and Representative Datasets: Training AI systems with balanced datasets to reduce bias.
- Algorithm Audits: Regularly evaluating models to identify and correct biased outputs.
- Inclusive Design Practices: Involving diverse teams in AI development to incorporate multiple perspectives.
Enhancing Efficiency
- Optimized Algorithms: Designing models to minimize computational demands while maintaining performance.
- Lifecycle Assessments: Evaluating environmental impact during development and deployment phases.
- Targeted Applications: Aligning AI solutions with specific, measurable goals to reduce waste.
Case Studies Highlighting Responsible AI in Action
Reducing Bias in Recruitment
A global technology firm implemented Responsible AI principles to revamp its hiring platform. By analyzing and correcting biases in training data, the platform improved its fairness and diversity outcomes, leading to more equitable hiring practices.
Enhancing Healthcare Outcomes
An AI-based diagnostic tool was redesigned with Responsible AI guidelines, ensuring it performed equally well across different demographics. This change led to more accurate diagnoses and increased trust among patients.
Environmental Sustainability
A leading AI research lab optimized its model training processes to reduce energy consumption. By focusing on energy-efficient algorithms, the lab significantly lowered its carbon footprint without compromising performance.
The Role of Stakeholders in Promoting Responsible AI
Developers and Engineers
- Conducting thorough data audits.
- Building explainable AI models to enhance transparency.
Organizations and Businesses
- Establishing clear AI governance policies.
- Allocating resources for regular algorithm reviews.
Regulators and Policymakers
- Creating legal frameworks to enforce Responsible AI practices.
- Encouraging cross-industry collaboration for ethical AI standards.
Why Responsible AI Matters
Responsible AI is not just a technical necessity; it is a moral imperative. By addressing biases and inefficiencies, it ensures that AI systems:
- Foster Trust: Building user confidence in AI technologies.
- Promote Inclusivity: Creating equitable opportunities for all.
- Drive Sustainable Progress: Aligning innovation with environmental and societal goals.
Conclusion
Responsible Artificial Intelligence offers a roadmap for addressing the challenges of bias, inefficiency, and ethical ambiguity in artificial intelligence. By integrating fairness, transparency, and accountability into AI systems, we can harness their potential responsibly and effectively. It is only by taming the machine that we can ensure AI remains a tool for progress, innovation, and the greater good.