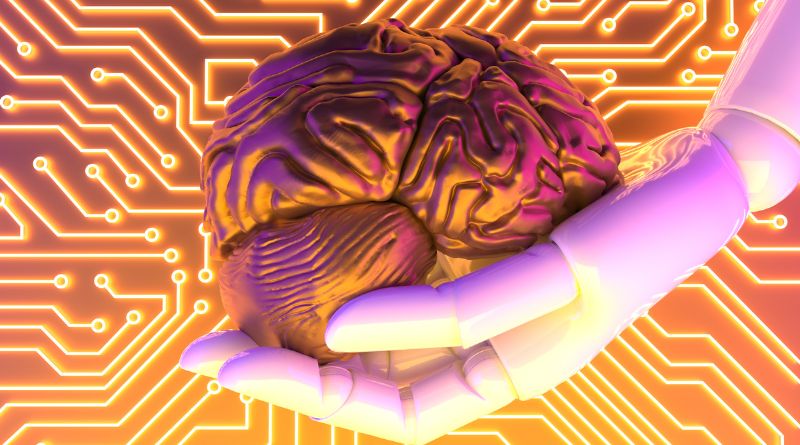
Artificial intelligence (AI) has become an undeniable force in our world, from revolutionizing healthcare diagnostics to streamlining business operations. However, with this immense power comes significant responsibility. Biases in AI systems can lead to discriminatory outcomes, while poorly designed algorithms can exacerbate existing inefficiencies. This is where the concept of responsible AI emerges – a framework for developing and deploying AI with human well-being at its core.
Dismantling Bias: The Achilles’ Heel of AI
One of the most pressing concerns surrounding AI is bias. AI systems learn from data, and if that data reflects societal prejudices, the algorithms will inherit them. Imagine a loan approval system trained on historical data that favored male applicants. Even if unintentional, such bias can have devastating consequences, perpetuating social inequalities.
Responsible AI tackles this challenge head-on. Here’s how:
Data Diversity: Responsible AI emphasizes using diverse and representative datasets. This ensures the algorithms are exposed to a wider range of experiences, reducing the chance of perpetuating existing biases.
Algorithmic Fairness: Techniques like fairness-aware model selection and counterfactual analysis can identify and mitigate bias within the algorithms themselves.
Human Oversight: While AI offers automation, responsible development doesn’t eliminate human judgment. Human experts can review AI decisions and intervene if bias is suspected.
By implementing these practices, responsible AI can ensure AI systems don’t amplify existing inequalities but instead promote fairness and inclusivity.
Beyond Bias: Mitigating the Risks of AI
Bias is just one piece of the puzzle. Responsible AI also addresses broader risks associated with AI deployment.
Explainability and Transparency: Many AI systems operate as black boxes, making their decision-making process opaque. Responsible AI advocates for explainable AI (XAI) techniques that allow humans to understand how AI arrives at its conclusions. This transparency fosters trust and helps identify and address potential issues.
Security and Robustness: AI systems can be vulnerable to manipulation or hacking. Responsible AI emphasizes robust algorithms resistant to adversarial attacks and data poisoning. This ensures the integrity of AI systems and protects against malicious actors.
Privacy and Security: As AI interacts with vast amounts of data, privacy concerns arise. Responsible AI focuses on data security, minimizing the collection and storage of personal information, and ensuring user consent is obtained and respected.
These considerations are crucial for ensuring AI serves humanity, not the other way around.
Case Studies: Responsible AI in Action
Theoretical frameworks are essential, but real-world examples showcase the power of responsible AI.
Fairness in Facial Recognition: Facial recognition technology has faced criticism for racial bias. Companies like IBM are developing fairer algorithms by using more diverse datasets and implementing bias detection techniques.
AI for Environmental Sustainability: AI can analyze environmental data to identify climate threats and optimize resource management. Responsible AI ensures such systems consider social and ethical implications – for instance, ensuring AI-driven resource allocation doesn’t disadvantage certain communities.
AI in Healthcare: AI has the potential to revolutionize healthcare diagnostics and treatment. Responsible AI ensures these systems are fair, unbiased, and prioritize patient well-being over profit margins.
These examples demonstrate how responsible AI can be harnessed for positive change across various sectors.
The Road Ahead: Building a Responsible AI Future
The development and deployment of responsible AI requires a multi-pronged approach.
Collaboration: Collaboration between developers, policymakers, and ethicists is crucial. Developers need to design with fairness and safety in mind; policymakers can establish regulations; and ethicists can guide the development process.
Public Awareness: Public education is essential for building trust in AI. People need to understand the capabilities and limitations of AI to participate meaningfully in shaping its future.
Continuous Improvement: As AI continues to evolve, so too should responsible AI practices. Regular audits and assessments of AI systems should be conducted to identify and address emerging risks.
Building a responsible AI future is a continuous process that requires commitment from all stakeholders. By working together, we can ensure that AI serves humanity as a powerful tool for good, not a source of unintended harm.